Understanding the AI Safety Index
Definition of the AI Safety Index
The AI Safety Index is a comprehensive tool designed to assess the safety practices of leading artificial intelligence (AI) companies. Developed by the Future of Life Institute, it aims to provide a structured evaluation of how well these companies manage risks associated with their AI technologies. This index evaluates various dimensions of AI safety, including risk assessment, governance, and transparency, ultimately serving as a benchmark for responsible AI development.
Purpose and Goals of the AI Safety Index
The primary purpose of the AI Safety Index is to enhance accountability within the AI industry. By publicly grading companies, it seeks to promote a culture of safety and responsibility in AI development. The goals include:
- Encouraging Transparency: By providing insights into safety practices, the index fosters openness among AI developers.
- Identifying Risks: It highlights areas where companies may be falling short in their safety measures, thereby motivating improvements.
- Guiding Policy: The index serves as a reference for policymakers to understand the current landscape of AI safety and inform regulatory frameworks.
Key Organizations Behind the AI Safety Index
Future of Life Institute: Role and Contributions
The Future of Life Institute (FLI) is a nonprofit organization focused on mitigating existential risks posed by powerful technologies, particularly AI. FLI plays a pivotal role in the AI Safety Index by assembling a panel of independent experts who evaluate the safety practices of AI companies. Their commitment to promoting safe AI development is evident in their ongoing research and advocacy initiatives.
Independent Review Panel: Composition and Expertise
The AI Safety Index is graded by a panel of seven independent reviewers, consisting of prominent figures in AI research and ethics, including:
- Stuart Russell: A leading expert in AI safety and ethics.
- Yoshua Bengio: A Turing Award winner known for his contributions to deep learning.
This diverse panel ensures that the evaluations are comprehensive and informed by a wide range of expertise.
Collaboration with Regulatory Bodies
FLI collaborates with various regulatory bodies to align the AI Safety Index with emerging standards for AI safety. This collaboration enhances the credibility of the index and ensures that it reflects the latest developments in AI governance.
Grading Methodology of the AI Safety Index
Categories of Evaluation
The AI Safety Index evaluates companies across six key categories:
- Risk Assessment: Measures how effectively a company identifies and mitigates potential risks associated with its AI systems.
- Current Harms: Assesses the impact of existing AI technologies on society.
- Safety Frameworks: Evaluates the robustness of safety protocols in place.
- Existential Safety Strategy: Considers strategies for managing the risks associated with advanced AI.
- Governance and Accountability: Examines the governance structures that ensure safety practices are upheld.
- Transparency and Communication: Analyzes the clarity and openness of a company’s communication regarding AI safety.
Scoring System Explained
The scoring system uses a letter grade format (A to F) based on the performance in each category. For instance, a company may receive an "A" for excellent risk assessment practices but a "D" for transparency, leading to a composite score that reflects its overall safety posture.
Recent Findings from the AI Safety Index
Overview of Company Grades
The inaugural AI Safety Index report revealed concerning grades for major AI companies:
- Anthropic: C (highest overall grade)
- OpenAI: D+
- Google DeepMind: D+
- Meta: F (lowest grade)
These grades highlight the urgent need for improved safety measures across the industry.
Common Weaknesses Identified
The report identified several weaknesses prevalent among AI companies:
- Vulnerabilities to Adversarial Attacks: All companies showed susceptibility to various forms of cyber threats.
- Lack of Robust Safety Frameworks: Many companies could not demonstrate comprehensive safety protocols.
- Inadequate Existential Safety Strategies: Most companies failed to articulate effective strategies for managing the risks associated with advanced AI.
Importance of AI Safety Metrics
Why Safety Metrics Matter for AI Development
Safety metrics are crucial for guiding AI development, ensuring that technologies are not only innovative but also reliable and secure. They provide benchmarks for companies to evaluate their safety practices against industry standards.
Real-World Implications of Poor AI Safety Standards
Inadequate safety standards can lead to significant real-world consequences, including:
- Harm to Individuals: Flawed AI systems can result in unfair treatment or discrimination.
- Societal Risks: Uncontrolled AI technologies may pose threats to public safety and security.
- Legal Repercussions: Companies could face lawsuits or regulatory penalties for failing to adhere to safety standards.
The Case for Regulatory Oversight in AI
Given the rapid advancement of AI technologies, there is a compelling case for regulatory oversight. A structured approach to AI governance, akin to what exists in the pharmaceutical industry, could help ensure that AI technologies are developed responsibly.
Comparative Analysis of AI Safety Standards
Differences Between AI Safety Frameworks
Two prominent AI safety frameworks include:
- ISO/IEC 42001: Focuses on establishing a comprehensive management framework for AI.
- NIST AI Risk Management Framework: Emphasizes a structured approach to managing AI risks with flexibility for organizations.
International Perspectives on AI Safety Regulation
Regulatory approaches differ significantly across regions:
- EU AI Act: Implements a risk-based approach with stringent compliance requirements.
- U.S. AI Regulatory Frameworks: Currently fragmented, relying on existing laws but moving towards new national standards.
Lessons Learned from Industry Practices
The AI Safety Index underscores the importance of learning from industry practices. Companies that adopt best practices in safety and transparency are more likely to foster public trust and mitigate risks.
Future Directions for AI Safety Standards
Proposed Improvements to the AI Safety Index
Future iterations of the AI Safety Index may include expanded criteria for evaluation, ensuring that it remains relevant as AI technologies evolve. Incorporating feedback from industry stakeholders will also enhance its effectiveness.
The Role of Stakeholders in Enhancing AI Safety
Stakeholders, including tech companies, regulators, and civil society, must collaborate to strengthen AI safety. Open dialogue and shared responsibility will be essential for developing effective regulatory frameworks.
Long-Term Vision for AI Safety Metrics and Standards
The long-term vision for AI safety metrics involves establishing universal standards that can guide responsible AI development globally. This would require international cooperation and commitment to ethical principles in AI.
Conclusion
Summary of Key Insights
The AI Safety Index provides a crucial framework for evaluating the safety practices of leading AI companies. The findings reveal significant gaps in safety measures, emphasizing the need for improved accountability and transparency in the industry.
The Path Forward for AI Safety and Governance
As AI technologies continue to advance, establishing robust safety standards will be paramount. Collaboration among stakeholders, alongside effective regulatory measures, will help ensure that AI develops in a manner that prioritizes public safety and ethical considerations.
Key Takeaways:
- The AI Safety Index evaluates leading AI companies across six key categories.
- Anthropic received the highest grade (C), while Meta received an F, indicating significant safety gaps.
- Common weaknesses include vulnerabilities to adversarial attacks and inadequate safety frameworks.
- Regulatory oversight is essential for ensuring responsible AI development.
- Future improvements to the AI Safety Index will focus on expanding evaluation criteria and stakeholder collaboration.
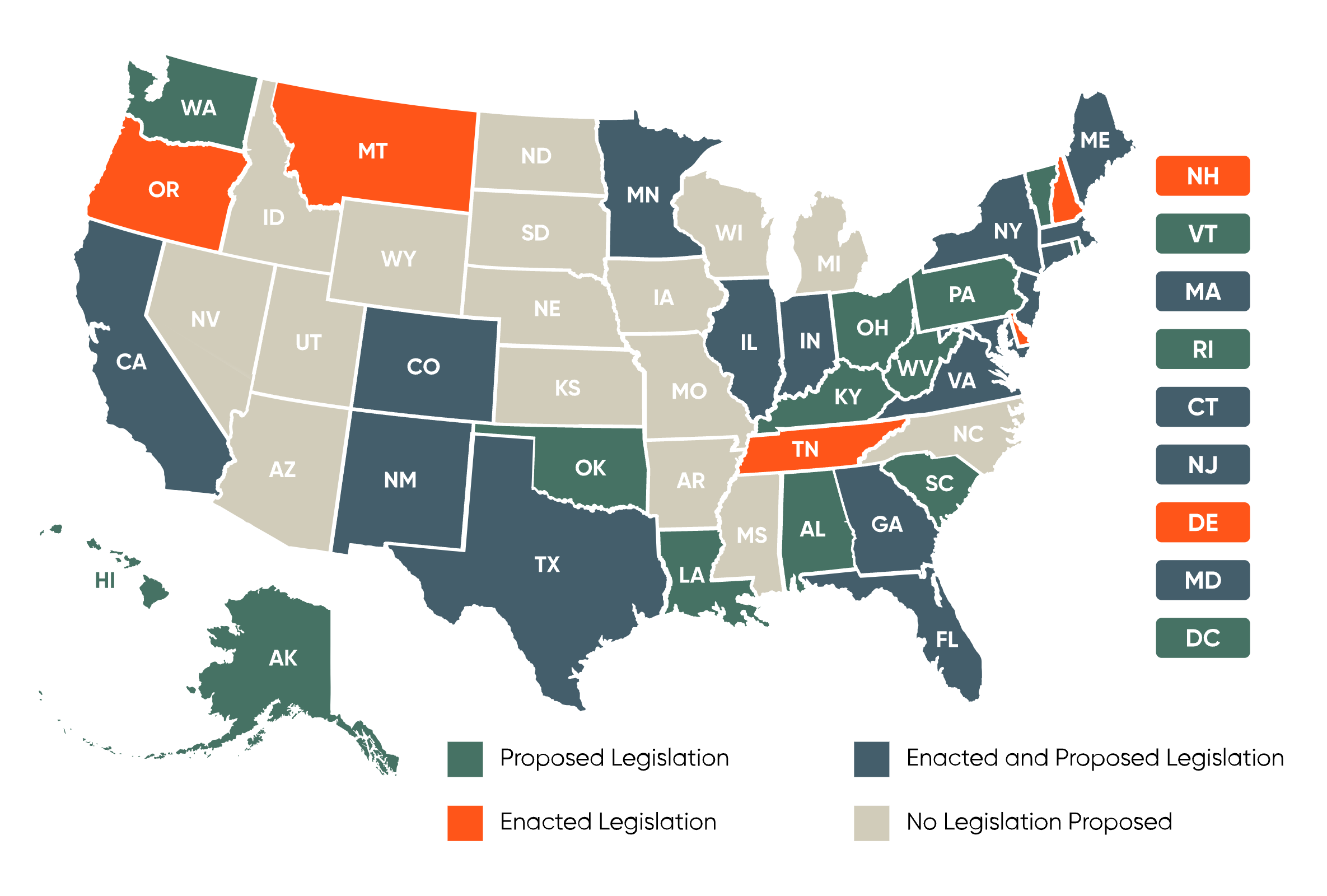